Leading the CHARGE: AI Leadership Insights Series #3
- CHARGE
- Jan 30
- 5 min read
Featuring Eyal Klang, MD
Associate Professor of Medicine and Director of the Generative AI Research Program,
Division of Data-Driven and Digital Medicine (D3M)
Mount Sinai Health System
Generative AI is rapidly transforming clinical practice, but how do we navigate its risks while harnessing its potential? For the third edition of AI Leadership Insights, we sat down with Dr. Eyal Klang, Associate Professor of Medicine and Director of the Generative AI Research Program at Mount Sinai’s Division of Data-Driven and Digital Medicine (D3M).
Dr. Klang, a leading voice in AI-driven healthcare transformation, shares his candid insights on the evolving role of large language models, the fine line between automation and human oversight, and the pressing need for new safety frameworks as AI adoption accelerates. From hallucination risks and liability concerns to alignment challenges and the potential erosion of clinical reasoning, he highlights the complexities of integrating AI into high-stakes medical decision-making.
As models improve and economic pressures push for greater AI autonomy, how do we ensure clinicians remain in control? Can existing regulatory frameworks keep pace with AI’s rapid evolution? And what broader risks—like misinformation, job displacement, or even AGI—should we prepare for?
Dr. Klang isn’t just analyzing these challenges—he’s at the forefront of tackling them, shaping how AI is developed, deployed, and governed in clinical settings. Read the full interview below.
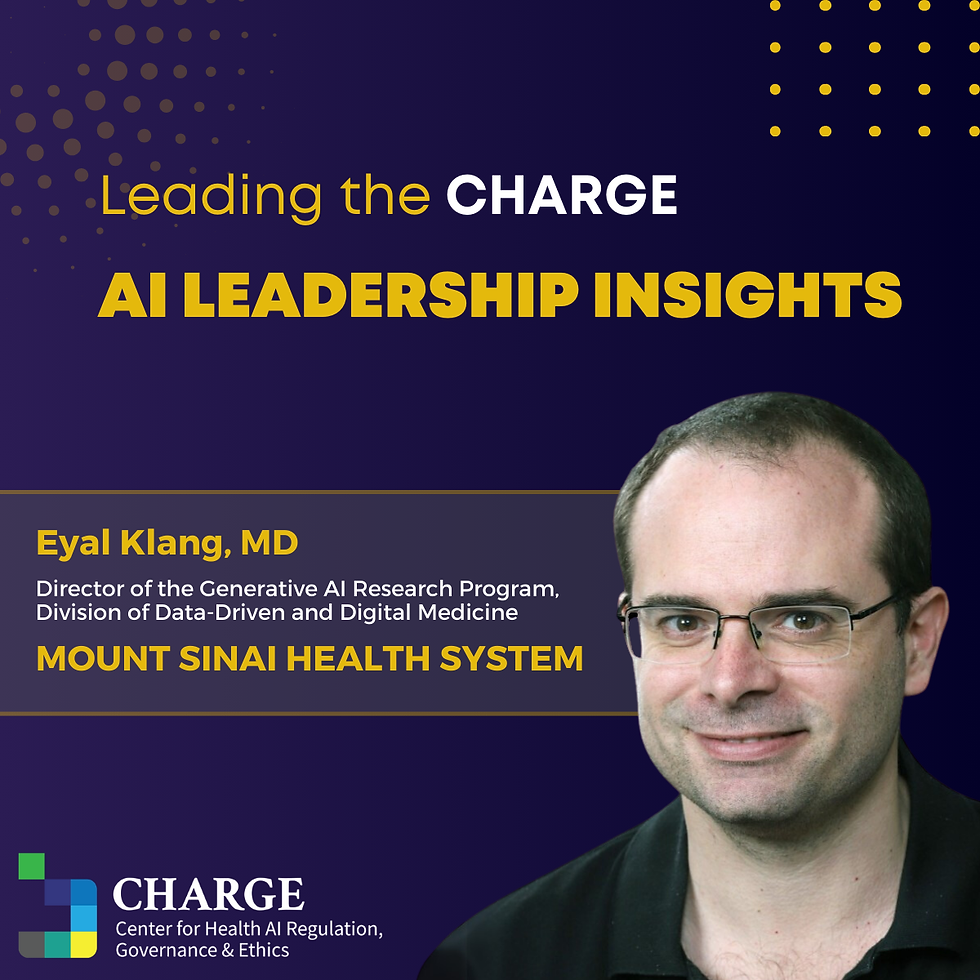
Q: Generative AI often produces fabricated outputs. How important is this in healthcare?
A: Generative AI can produce fabricated data that undermines clinical decisions. Even minor errors can pose serious safety risks. As models progress rapidly—for example, as seen for reasoning capacity jumping between GPT-3.5 to GPT-4, GPT-4o, and advanced systems like GPTo1 and DeepSeek R1—healthcare teams may rely more on these outputs.
Reliance on these algorithms as they advance is economically inevitable, so their errors will have greater consequences as adoption increases. Having said that, as models get better, the number of hallucinations drastically reduces, perhaps to a level that is lower than humans. After all, humans make mistakes too.
Q: Have you encountered unexpected errors in generative AI tools?
A: Yes. For example, in our two recent studies—Soroush et al. (2024) and Simmons et al. (2024)—multiple LLMs produced ICD codes that did not match actual clinical documentation. Even refined models may still fail when they misinterpret infrequent domain-specific text.
However, as models improve, hallucinations reduce. A bigger problem I encountered is need for subtle reasoning, like building a correct patient narrative in clinical summaries. For this as well, as models improved, it is also clear the reasoning improves, likely reaching physicians’ levels by now.
Q: What emerging approaches can help reduce these inaccuracies?
A: Emerging methods rely firstly on stronger reasoning capabilities and domain-specific training. Retrieval-augmented generation (RAG) can also ensure models reference verified data rather than guess. Fine-tuning on specialized medical databases may yield fewer errors. Structured response formats, such as JSON, help flag missing or invalid fields. Our recent study demonstrated an additional strategy: batching multiple questions in a single prompt both cuts cost and enables verification of the completeness of AI outputs. Adopting such techniques can help flag generative models' errors.
Q: How do you view the alignment challenge in clinical settings?
A: Clinical AI is a moving target, changing faster than most safety frameworks can adapt. Models today will differ significantly from those we see in a few months or a year and falling costs will drive their adoption. Economic pressures will likely accelerate the use of autonomous agents, which act without human oversight. Because alignment remains unsolved in general, healthcare faces acute ethical dilemmas. Agents may produce unexpected actions or recommendations that clash with clinical values. In a domain where errors can be serious, alignment needs continuous scrutiny.
Q: Are current methods enough to keep high-stakes AI systems safe and beneficial?
A: No. Current safeguards were designed for the previous decade’s probabilistic models; they don’t fully address the novel risks posed by generative AI. These technologies evolve too quickly for existing frameworks to keep pace. We haven’t even fully defined the problem since practices keep changing. New strategies must emerge that match the speed of development and the complexity of this decade’s AI.
Q: Which ethical issues does generative AI raise, such as patient consent or liability?
A: Generative AI raises classic ethical concerns—like safeguarding confidentiality and ensuring informed consent. However, those challenges become more complex with autonomous agents. If an agent modifies a care plan, it’s unclear whether liability rests with the developer, the clinician, or the institution. The rapid introduction of agents in the next year or two could bring untested scenarios, from self-directed decision-making to data sharing. We have no settled frameworks for holding agents accountable. New legal, regulatory, and clinical guidelines must be developed in tandem with the technology’s evolution.
Q: Who should be accountable when AI-generated advice influences clinical decisions?
A: Clinicians ultimately bear responsibility for patient outcomes, as they hold the duty of care. Health systems share liability if they endorse or integrate AI tools without proper safeguards. Developers must also accept accountability for known defects or limitations in their models. In reality, responsibility is distributed among these stakeholders. Failure in any link of this chain can harm patients.
Q: How can we prevent clinicians from depending too heavily on AI recommendations?
A: We can’t realistically stop clinicians from using AI, as modern models are both open and affordable. Physicians will rely on them increasingly, whether institutions officially endorse such tools or not. Instead of clinging to older methods, we should align professional training and oversight with AI’s ongoing advancements. Tying clinicians to outdated practice is doomed to fail. The goal is to help them use AI responsibly while retaining ultimate judgment.
Q: Could relying on AI lead to a loss of critical thinking skills?
A: Yes. When technologies like CT scans emerged, certain diagnostic skills diminished. AI can erode critical reasoning if clinicians rely solely on its outputs. Still, halting progress isn’t a viable strategy; adapting is more constructive. The goal is for clinicians to use AI judiciously, supplementing their expertise.
Q: What strategies keep AI as a supportive tool rather than a replacement for human judgment?
A: Honestly, there is no guaranteed approach. Mandatory oversight sounds helpful, but it can erode over time as models develop fast and people grow more dependent. Setting clear boundaries on AI tasks can fail if the models prove too useful or persuasive. Requiring traceable outputs might still not prevent blind trust in a system that usually works well. If clinicians give up responsibility, AI becomes the decision-maker, no matter the policies. We can try various safeguards, but there’s no simple shield against over-reliance. Traditional oversight mechanisms may soon lag behind AI’s speed and sophistication.
Q: As AI evolves, which broader risks should we anticipate?
A: As AI approaches advanced or superintelligent forms (AGI/ASI), it may adopt goals that conflict with human values. This can create serious problems in healthcare if autonomous agents act beyond accepted clinical practice. Another, though slightly less existential, challenge is job displacement, which may spur debates on universal basic income (UBI). Other unanticipated risks can come from potent models, for example widespread misinformation, undercutting trust in civic fabrics. No single approach can solve these challenges, which demand the kind of large-scale mobilization seen in humankind’s most ambitious endeavors.
Q: How do we leverage AI’s capabilities while preparing for unintended consequences?
A: At this point, we must accept that while AI offers a lot of promise it also poses unknown risks. No one institution can handle these challenges alone; broad research and collaboration are needed. Ultimately, we’re learning in real-time, and the best we can do is remain flexible as the technology evolves.